Show Notes
In this episode, Amith and Mallory explore how AI is impacting the scientific method, from hypothesis generation to experimentation and data analysis. They discuss Robin AI, an AI-powered legal tool, and highlight the importance of associations preparing for or even creating their own industry-specific AIs. Finally, they discuss the New York Times' lawsuit against OpenAI and Microsoft, delving into the challenges of AI-generated content and its potential conflicts with copyright laws.
- Let us know what you think about the podcast. Drop your questions or comments in the Sidecar community: https://community.sidecarglobal.com/c/sidecar-sync/
- Join the AI Learning Hub for Associations: https://sidecarglobal.com/bootcamp
- Download Ascend: Unlocking the Power of AI for Associations: https://sidecarglobal.com/AI
- Join the CEO AI Mastermind Group: https://sidecarglobal.com/association-ceo-mastermind-2024/
Thanks to this episode’s sponsors!
- AI Learning Hub for Associations: https://sidecarglobal.com/bootcamp
Tools/Experiments mentioned:
- Elicit: https://elicit.com/
- Getty Image Generator: https://www.gettyimages.com/ai/generation/about
- Robin AI: https://www.robinai.com/
Topics/Resources Mentioned:
- Eric Schmidt: This is how AI will transform the way science gets done: https://www.technologyreview.com/2023/07/05/1075865/eric-schmidt-ai-will-transform-science/
- Robin AI’s legal copilot gets $26M venture infusion: https://siliconangle.com/2024/01/02/robin-ais-legal-copilot-gets-26m-venture-infusion/
- New York Times sues Microsoft, ChatGPT maker OpenAI over copyright infringement: https://www.cnbc.com/2023/12/27/new-york-times-sues-microsoft-chatgpt-maker-openai-over-copyright-infringement.html
Social:
- Follow Sidecar on LinkedIn: https://www.linkedin.com/company/sidecar-global
- Amith Nagarajan: https://www.linkedin.com/in/amithnagarajan/
- Mallory Mejias: https://www.linkedin.com/in/mallorymejias/
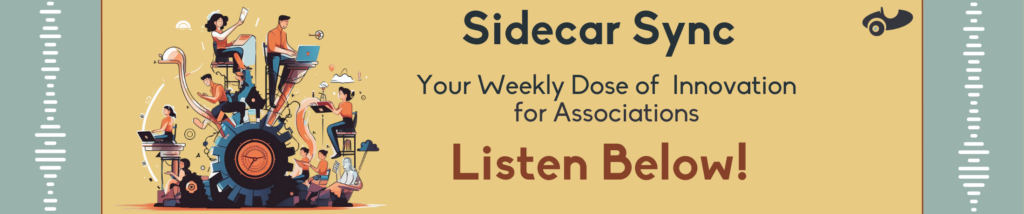
Amith Nagarajan: [00:00:00]
Hi, everybody and welcome to the latest episode of Sidecar Sync. It is now 2024, and we're excited to be back with the fresh new episode covering a lot of interesting ground in the world of AI and associations. [00:01:00] But before we get started, I’d just like to ask that if you are enjoying this podcast, please consider sharing it with your friends on social and possibly leaving a review that will help us grow the audience and reach more people that we can help with this content.
Also, I'd like to give thanks to our sponsor Sidecars AI Learning Hub. The AI Learning Hub is a learning journey. It is all about bringing your team together to learn the latest in AI and how it applies to the association world. It's not just about AI for AI's sake, but it's specifically tailored to the association world.
Use case after use case after use case explaining to you how AI can be put into practice in a reasonable. Easy, accessible way in your association access to the boot camp gives you 12 months of continual evolving lessons as well as weekly live office hours. So consider the AI Learning Hub as a way of accelerating your own learning journey [00:02:00] as well as bringing your team together.
More information is available at sidecarglobal.com/bootcamp.
Mallory Mejias: Our first episode of the New Year, Amith, how were your holidays? How was your New Year?
Amith Nagarajan: They were fantastic. A lot of great time with family time to unplug just a little bit from all the craziness that we encounter every week of 2023. So it's nice to start fresh and have a great time. How about you?
Mallory Mejias: Yeah, I had a good time as well. I took a little bit of time off. I took a trip to Georgia as well, which was pretty fun. I wasn't sure if I was going to return to a mountain of AI news. I feel like actually not that much has happened in the past few weeks. Do you agree? Or maybe I've just missed some of it.
Amith Nagarajan: Things have definitely been calmer from my perspective. You know, I think there's been less news. It's probably smart for a lot of the companies that are out there that have pending things to share to hold off until people get back so that they're not losing attention. But yeah, it's definitely been quieter the last couple weeks and I don't expect that to [00:03:00] last.
Mallory Mejias: I agree. I think maybe there was just this slight lull and we will be back in action in the coming days. Our first topic for today is the intersection between AI and science, which we've talked about a bit on this podcast before, but specifically the intersection of AI and the scientific method, which is an interesting take.
Eric Schmidt, Google's former CEO, shared a compelling vision on how AI is set to revolutionize the field of science. He highlighted several groundbreaking applications of AI that are already making significant strides. For instance, NVIDIA's AI powered digital twin of the planet called Earth 2 uses a model to predict weather patterns with unprecedented speed and accuracy.
This model can generate thousands of predictions, which far surpasses traditional weather forecasting methods and is crucial for predicting rare but deadly disasters. Beyond climate modeling, AI models have been instrumental in identifying new antibiotics, controlling plasma and nuclear fusion reactions, and even in [00:04:00] radiology with FDA approved devices.
But, aside from these individual advancements, AI has the potential to transform the way that we do science from the ground up, which I think is pretty interesting. So, if we go all the way back to grade school, college maybe, thinking about the scientific method, starting with hypothesis generation. AI has the ability to analyze vast datasets, which enables it to identify patterns and correlations that might elude human researchers.
Tools like Paper QA and Elicit already use LLMs to scan databases of articles to provide summaries of existing papers. This data aids in formulating stronger, more informed hypotheses to test. We all know after you have the hypothesis, we go into the experiment, experimentation phase. AI accelerates experimentation by simulating outcomes, predicting results, and even suggesting optimal experimental conditions.
This ultimately reduces time and resources that might be spent on trial and error. Once we run [00:05:00] the experiment, it's time to do data analysis, and AI certainly excels at this part. This is crucial in fields like genetics or climate science where data, where data sets are vast and complex, AI can uncover insights from data far more rapidly than traditional methods.
I don't know about you Amith, but I remember many a day in, in univariate statistics in college. I studied psychology, so I am very thankful that AI can do some of the data analysis for sure.
Amith Nagarajan: Definitely.
Mallory Mejias: And then finally, in terms of peer review and replication, I found an interesting stat on this topic that around 70 percent of scientists report having been unable to replicate another scientist experiment.
AI can assist in the peer review process by checking for inconsistencies or errors in research, and it can also lower the cost and effort of reproducing experiments. So we can kind of see that every step of the way, every step of the scientific method, AI can be used to enhance the steps, but I also want to add that [00:06:00] there's this idea that in the future, we might actually need little human intervention in this process with something known as self driving labs, and these are automated robotic platforms that are combined with AI to go through the steps of the scientific method, with little to no human interaction, potentially.
Amith, what are your initial thoughts on AI and the scientific method?
Amith Nagarajan: Well, first of all, on the weather forecasting piece, I think that's going to be exciting for all of the big reasons on earth in terms of forecasting weather changes and disasters and how that will affect. Quality of life and safety. I'm particularly excited about that because I'm a skier and knowing when snow is going to fall is something I'm pretty pumped about.
So we don't tend to have great insight into that. And I think that weather forecasting is a perfect example where it's a different approach and the scale of data that AI is able to deal with and the complexity and the scenarios that it's able to evaluate are quite fascinating. This idea you mentioned earlier about the digital twin, [00:07:00] essentially, for those that aren't familiar with that term, it's basically a simulation.
It's a way of saying, hey, I have a digital twin of a person or a system like the global weather patterns. You might have a digital twin of a company that represents that organization’s inner workings of all the people in the company, the departments, the processes. That's really what digital twins tend to mean.
The concepts been around for a while, but with AI. We have the possibility of creating these really you know, incredible simulations through these digital twins for a lot of different things. So that's really fascinating. So you might say, oh, well, I want to have a digital twin of my company and simulate what a different org structure might look like and how my people react to it.
What would that do to the culture? And how would that affect decision making? And how would that affect speed? Really interesting stuff. Broadening the conversation back to the topic, though, transformative impact on science specifically. I think that it's super interesting how you broke it down, Mallory, into the different elements or steps in the scientific [00:08:00] method from hypothesis generation through peer review.
And when you think about it that way, it's like the tool set we already have can do a lot of those things, right? It can't be on autopilot yet, but it can be a really significant assistant, and that's exciting. And where we're heading with, you know, what you described as self-driving labs, particularly the mixture of robotics with AI could really accelerate things further.
You know, some of the topics we've discussed in past episodes, like, for example, the idea of AI agents and multi-agent frameworks like auto-gen could be really instrumental in being able to stitch together these different steps of the scientific method and accelerate the processes. I think it's speed that's interesting, but it's also the ability to do lots more parallel experimentation.
So if we're talking about a field like drug discovery, for example. If we want to be able to hypothesize and then experiment. on a much larger array of potential molecules that might be, you know, targeting [00:09:00] particular diseases. Well, if we can only do that with 10 or 50 or 100 compounds or molecules, that is limiting because, first of all, as you mentioned, people are biased and we have natural inclinations to focus on the things that we obviously feel have the most promise.
But we overlook a lot of potential opportunities because we simply can't compute at the scale of what we're now talking about. So, by having those additional opportunities and being able to run many more experiments in parallel, I think that's gonna lead to some groundbreaking scientific discovery really novel innovation in, in all fields of science.
So, my answer is, I'm really excited about it. I think there's a lot that's gonna happen this year. With scientists deploying these technologies in part, and then eventually that idea of a self driving lab, those concepts are coming together already. It's going to be a real thing. It's going to be very powerful.
Mallory Mejias: It made me laugh a bit when you said you were a fan of the digital twin earth model because immediately, I was [00:10:00] thinking, oh, obviously because of the hurricanes, because if you all don't know, Amith and I both primarily reside in New Orleans and no, of course, Amith, you were, you were thinking skiing all the way.
Amith Nagarajan: It's all about the snow for me. Of course, I deeply care about the regions that are affected by extreme weather like New Orleans and hurricanes, but you know, that's really the most exciting aspect of this, of course, in day to day life. I think it's really helpful to have a good weather forecast.
And you know, if you're an outdoor enthusiast, of course, having a good idea of of climate is really, really helpful.
Mallory Mejias: Absolutely. Walking through this research that I did and going through the steps just now on the podcast, I was really thinking that pretty much every step of the way from hypothesis generation, experimentation, data analysis, and peer review and replication, you could make the argument that AI right now or eventually will outperform humans in each of these steps, especially with science particularly, which is so much about trying to remove human error from processes. [00:11:00] So with that, well, one, do you agree with that statement? And then two, it's a little scary because if that is the case, where does this leave human scientists?
Amith Nagarajan: You know, I wouldn't be able to provide an expert opinion on that in the sense of, like, what's happening in real scientific environments. And, you know, will the AI potentially replace entirely what the human does. But I suspect it will be an answer very similar to what we've discussed. In other fields where it's not so much AI or human, but rather it's the AI plus the human and the idea of what we do as people in combination with AI is going to evolve. So I think that the scientists will still be involved in overseeing the process in coming up with a lot of the problem set that we're trying to go solve for. I think where the AI will be able to help tremendously is the scale at which AI can, you know, “think” and be able to suggest ideas to solve problems. That's that are potentially things that humans get stuck on. [00:12:00] So I think that every field is going to adapt. Medicine is going to adapt. Law is going to adapt.
Accounting is going to adapt. The roles I think that people will play will be different. But ultimately, these tools are going to open up a completely different paradigm. We're gonna be able to think about all this stuff differently. Instead of having to wait ten years for a new drug, the cycle time to go from idea to drug approved by the FDA might be three years, or something that sounds completely crazy, or maybe even less than that, you know, maybe it's six months.
So I think those opportunities exist in the next decade or two to radically change a number of, like, deeply impactful areas of science. I think that's also true when it comes to renewable energy, you know, so much fundamental work has to happen around physics and material science to really scale renewables and to scale portability and energy density and batteries.
And we've talked about that a little bit in the podcast and the material science episode where we talked about how new materials might affect some of these grand [00:13:00] challenges in these disciplines. So, to me, it's an opportunity to solve for more as opposed to, oh, it's a cost savings. Of course, there's going to be that in every field, but it's more about how do we go and achieve tremendously more than we ever thought would be possible.
Mallory Mejias: Right, I think that's where my mind is going as well. I like this idea of AI plus human, but of course, thinking in the traditional context, the context that we know right now to be the world, it seems like if we have AI plus humans, We'll just need less humans to do the same amount of work, but you're saying what I'm hearing is that there will just be new types of work, new paradigms that we can't even really fathom right now because they don't exist.
Amith Nagarajan: Well, you know, we as a species think in linear terms. Through the process of evolution, you know, we look for our next meal, and that's just how it is. Like, we're hardwired that way biologically. You know, we look to have vision of various flavors, and some people are really good at that, and some people are not so great at that, but, you know, we tend to be very linear or sequential in the way that we process the world, and this is an exponential time.
These [00:14:00] are things that are changing at such a crazy rate, it's hard for all of us to really consume the information and understand it really thoroughly. So my way of thinking about it is it's kind of simplistic on the one hand, but I think is perhaps a good way of looking at it, which is to say, okay, if we imagined the AI. Was a person and we just had, you know, 10 more employees or 100 more employees that perhaps didn't have the breadth of skill sets of our best employees who could do everything but had certain limited subsets of skills were good at particular sub disciplines, right? How do we organize those people to get work done?
I think part of what this topic is touching on in the field of science is applicable more broadly to everyone, which is you know, how do you experiment in life and in business? How do you take a process and tweak it and see what happens? You know, we spend so much time, for example, debating is this the best way to do this particular thing?
It might be like, oh, this is the way we're going to review and approve papers for submission to a [00:15:00] journal or speakers for a conference. And we've done it this way for a long time. What would happen if we did this way? Well, this idea of digital twins potentially could be a way of simulating that and seeing what would happen in those scenarios or just obviously easily being able to experiment in in real life too.
Mallory Mejias: Well, that's exactly to what my next question was going to be was about extrapolating this information from science and asking what it would look like to run experiments within our own organizations and specifically the self-driving labs. Potentially, I imagine an example where maybe is analyzing member engagement across all your platforms, and then you ask it to run different simulations, maybe for a website update to see what that looks like.
What happened after that or a change in your newsletter schedule to simulate changes in member engagement. What do you think about that?
Amith Nagarajan: No, I think you're on the right track with that. And, you know, we talk a lot about A/B testing and marketing where we have perhaps two different homepages or two different videos that we want to test with our audience. And I think that's going to continue to be a really good tool set to [00:16:00] basically see and experiment, you know, with the actual live audience.
But what if you could simulate an audience for your website? And to your point, right where you could say, hey, effectively, you have this digital twin of the website, which also includes the digital twin of the audience. And you kind of look at that behavior of that audience historically and more broadly at large on the Internet and think about what's going to likely happen in a simulation with a particular flavor of the website that you put out there.
And then perhaps you still do A/B testing with a live audience, but the simulation could help you make good decisions that lead to a better experience online. I think that's one of many examples that are fantastic. So I really like what you said there. I would encourage associations to think about this discussion in two ways.
First, you know, we've explored the impact of AI on a variety of fields in this podcast, and we'll continue doing that because I think they're just both interesting and also informative in terms of what that means for an association in that field. So if you're an association anywhere in science or [00:17:00] engineering anywhere in that general vicinity, this is what's happening to and for your members. And so you have to be aware of it. You have to be in a leadership role where you're helping them figure this stuff out. So that's part of how associations need to process this. The other part of it is, of course, the way you're bridging to now, which is how do you think about this type of innovation in the context of an internal process and how do you apply it to your organization?
And there's many ways of getting inspired from adjacent fields or even totally different fields and applying them to what you do day to day.
Mallory Mejias: Amith, do you think we have the tools currently to create a digital twin of a website and then a digital twin of an audience and run those simulations? Maybe not the public, but do they exist?
Amith Nagarajan: Yes, the fundamental building blocks are there. So what I consider kind of the native elements that you need to use to construct that outcome, that solution are there. The accessibility and the cost of doing it at the, to the level we're talking about [00:18:00] would be unreachable for most at the moment.
But like everything else that's happening, you know, we have this six month doubling of performance relative to cost, right? So we talk about the six month doubling of AI. It simply means that the capabilities you get for a dollar today, you're gonna get twice as many capabilities for that same dollar six months from now.
And so what that also means is in the context of your question, yeah, even if something is ridiculously expensive to do today, very likely after a few of those doublings, it's going to become very affordable and the tooling around. It's going to keep getting better and better and better. You know, a good example that is video generation.
You know, we've talked about HeyGen, and we've talked about other tools in this podcast. We've demonstrated them through the AI Learning Hub and through a variety of other platforms over the last few months. And those tools are consumer grade. They're easy to use. They're not perfect, but they're pretty darn good.
And only a year ago, the equivalent type of tool set would have been quite expensive and difficult to put together. And so, again, it's this, this [00:19:00] process where you have to imagine where the world will likely be. Thinking about these capabilities, this is not science fiction, this is the near future. And so then thinking about, okay, if this digital twin type of idea could be put in place for my organization, or a portion of my organization, like my LMS or my website.
Let's talk about what that means 12 months from now. Let's start building towards that, even with testing different bits and pieces of it. And a scientist might say, well, this idea of the self-driving lab is really cool. Why don't I just use it to help me with data analysis? Why don't I just use it to help me with peer review?
Pick an element of what this fully encompassing solution might eventually include and go and play with that one piece. And those tools are definitely there and they're very accessible.
Mallory Mejias: Last question on that. Do you see A/B testing as something that could go away in the future? And I just mean that in terms of an organization deciding to run simulations only if they're highly accurate at predicting certain outcomes, as opposed to [00:20:00] actually running the test itself.
Amith Nagarajan: So when you're dealing with actual people, costs go up, speed slows down, and it's hard to do that at any significant scale. You think about like drug discovery, again, once you're in the latter and latter stages of the clinical trial process, you're dealing with massively increased costs and elongated timeframes because you're dealing with people in that context.
Obviously, you're dealing with safety as well as efficacy, but whenever you deal with people, things slow down and get expensive. And so, will you be able to radically reduce the number of actual empirical results you seek to gain from interactions with people? Yes. Will you completely eliminate the need for that?
I think perhaps so in some disciplines, but probably not in many others. Like, my personal bias for marketing would be, I might use a simulation environment to test a thousand different variations of my website. I'm probably still going to want to A/B test the top two or three ideas with actual people.
Even if the AI got really, really good, and it will get really, really good over the [00:21:00] next, say, five years to do something like that, I still think there's value in the feedback loop that comes from, you know, actual results dealing with and interacting with people. I think an argument could be made. That's the exact opposite of that, too.
But I think, you know, my biases are that the human feedback loop will still provide value, even with extremely advanced AI.
Mallory Mejias: Absolutely. Our next topic of today is RobinAI. In a significant development for AI in the legal sector, RobinAI, a company specializing in AI powered legal contract creation and review, has recently secured a substantial $26 million in a new funding round. This investment, which brings the total funding for RobinAI to nearly $43 million, marks a notable milestone in the integration of AI into legal processes.
The UK based firm, co-founded by a lawyer and a machine learning research scientists in 2019, utilizes Anthropic's Claude large language model, fine-tuned with a database of over [00:22:00] 2 million contracts. Robin AI's technology, available as a free Microsoft Word add in, enables users to swiftly create contracts, review existing ones using plain language prompts, and identify and propose edits.
This AI copilot significantly reduces the time required for contract reviews by over 80% and cuts costs by 75%. The success and growing popularity of RobinAI, underscored by its recent venture Capital Infusion, signal a broader shift towards a more tailored AI solution across different industries.
This trend holds particular significance for associations as these highly relevant industry specific tools will start to pop up more often and impact your members across sectors. Amith, I was impressed by this topic of the week and I also got an email in my inbox this morning actually from an AI newsletter.
I think it's called the AI Agenda, the one that you recommended to me. That's great for anyone listening about RobinAI. So, it seems to be a hot topic this [00:23:00] week. The idea of cutting contract reviews by 80 percent to me sounds wild because I imagine, you know, that's a billable thing that lawyers are doing.
It's just kind of, it's crazy to think about how much this has the potential to change that industry, but kind of pulling it out more broadly, what role do you see associations playing and helping their members adapt to changing landscapes in their professions because of AI?
Amith Nagarajan: Well, if there's a possible risk of offending some of our listeners, I don't think too many people will be disappointed by having fewer contracts to review. I know there's probably people out there who enjoy it, but I never have. I've looked at far too many contracts in my career and the idea of cutting contract review by a very, very large percentage makes total sense because so much of it is repetitive. So much of it is based on prior concessions that have been made in contracts and knowing what an organization is willing to agree to change, what they're not willing to agree to [00:24:00] change, which pieces of contract language are must have walk away from the deal kind of language versus the pieces that are a little bit more flexible.
Every company, every organization has those types of guidelines and it's kind of, you know, right now embodied primarily and the institutional knowledge really kind of in the brains of the people who do this stuff. Very rarely is it documented. But, you know, tools like this that are trained on the broader set of contracts that are out there and can also be tuned to understand your body of work in terms of the contracts in your organization.
It's an obvious use case in terms of leveraging current state-of-the-art technology, not even looking ahead at what's coming. So, I'm not surprised by this. I think it's super exciting. And I think that to your question of, you know, the role associations need to play. Let's talk about it from a few different angles.
So let's say that you are a state bar association and your members are attorneys in your state. And this is a tool that all of a sudden let's say you have attorneys that are a mixture of in house [00:25:00] counsel and people that are from outside firms who provide, you know, essentially billable hours to a variety of clients.
You know, for the folks that are in the outside role, this is a significant issue because their business model is based on hours. It's like paying for the ingredients, not the outcome. And so, you know, I'm not a big fan of business models like that in general because I like the idea of value based or outcome based pricing anyway.
But the legal industry is very much an hourly, billable hour type thing and tons and tons of other Businesses are like that. Actually, in many respects, the healthcare industry is like that. You pay by the service, which really boils down to labor and equipment. Not by the outcome. You don't pay to have a successful knee surgery.
You pay for all the different components that go into the knee surgery. Similarly, if I have litigation or a contract process or if I'm, you know, filing a patent, I pay for the bits and pieces that go into it rather than the outcome. And I think that's the thing that might be the shift that the industry gets forced into is to have value based pricing [00:26:00] or outcome based pricing in the legal sector where you go to your attorney and you say, listen, we want to file a new trademark for one of our new companies at Blue Cypress and we pay a flat fee, and that's a turnkey service to go from end to end to get the outcome we want, which is an approved trademark from the United States Patent and Trademark Office, as opposed to paying by the hour.
And in the context of contract work, it might be you pay your attorney a flat rate to get the contract done. So there is a shift in the business model that actually creates better alignment ultimately because the client and the attorney then are both achieving an outcome and the attorney is benefiting from the efficiency because if they get a flat dollar amount to achieve an outcome and they use these tools to cut out 80 percent of their labor they theoretically have more profit in there.
And in theory, the client is receiving the same amount of value. Of course, ultimately competition will drive pricing down. So it's an interesting question. I think associations need to be part of that discussion in their field. So once again, if you're a state [00:27:00] bar association or a national bar association or a specialty bar association in a particular field of law.
You need to be having these conversations with your members. You can't look at it from the viewpoint of something out of scope because it's a fundamental change that's going to happen in the industry. Whether you like it or not, I think the association must stand up and take a leadership role and, you know, help members figure out what the future looks like in the context of major changes like this.
So that's one side of it. The other side of it, of course, is what should the association be doing with this stuff to consider new products and services that the association itself potentially could offer to help members do these kinds of things? Because, you know, RobinAI and, you know, hundreds of other similar tools are going to pop up out of nowhere, and many of them will be heavily venture funded, and they'll sound great, and they'll probably provide utility.
But who knows how the technology is built, and who knows if it's a trustworthy source of content. One of the big things associations have is trust in their [00:28:00] brand. Another thing they have is a tremendous amount of trustworthy content. And so those are two ingredients that are necessary to spin up services like RobinAI.
So my question to association leaders is why shouldn't the association play in that game? Either directly or through perhaps a partnership with a technology company. Association should be thinking about how to leverage their intangible assets like brand, like content, like their network to potentially offer services like this to the market.
And that's radically different than what associations typically think about, but it's a natural extension in terms of how you help members be more successful is the fundamental mission of what most associations, you know, say that they're focused on.
Mallory Mejias: That's really interesting. I feel like we don't dive into that too much on the podcast. The idea of associations creating their own products and services. would, how would an association leader listening to the podcast go about doing that?
Amith Nagarajan: Well, you know, I think the first step is, is to allow [00:29:00] yourself to think this way. So rather than thinking your association is a collection of the 5 to 10 products and services you've been offering for the last 30 years, step back and reevaluate your purpose. You know, you exist in order to make a particular field more successful, to make a particular branch of medicine safer or more effective.
You know, you, you exist to serve that bigger purpose. The products and services that you currently offer are simply tools to help you achieve that goal. And so then when you think about it from that lens, you say, okay, well, how can these advancements in technology and AI help us accelerate that mission?
And in the context of these kinds of AI tools, imagine if the Bar Association said, hey, we have our own digital assistant. that is our Bar Association's certified assistant that has been trained on our content and is therefore, you know, probably going to be a lot higher quality than someone who doesn't have access to that repository of proprietary content.
Now you have to have a reason for thinking that you actually are going [00:30:00] to have a market advantage there. If you are a geographic association, perhaps there's a body of law in your region that you have really good insights into that nobody else does perhaps. Maybe you're a specialty association in a particular area, like intellectual property law or real estate law, and you have content that no one else has there or, you know, branching outside of the legal field and in various domains of health care or engineering, there's a lot of associations that have highly specialized content that they've compiled over decades in many cases, and a lot of it's evergreen.
And so you have to look at it and say, do you have a reason to think that you could be competitive in that space? Just because you're the association, that's not good enough. The brand strength is nice, but that by itself without a product that's differentiated is not going to help. So, you know, we talked about this a little bit in our book Ascend, Unlocking the Power of AI for Associations.
In that book, we have a chapter dedicated to the idea of a digital assistant, which is one idea for a new [00:31:00] product that could be offered by associations. members or to their audiences. And many associations would think of that and say, Oh, that's great. That's going to be an awesome member benefit. And that's okay.
Like it could be a member benefit, but you also need to think about perhaps modeling it as a multi-tiered offering where you're not just thinking about, oh, this is yet another thing I throw into the bag of value for membership. Perhaps that is the case for like a low level of service from the AI that you're providing or the tool you're providing.
But perhaps there's higher levels of tears. So we have some thought experiments around that in the book that I'd encourage you to get. It's freely available for download, by the way, for those that haven't heard. It's available at sidecarglobal.com/ai. And you can download the pdf of the book for free.
Share it with everyone. Like in the chapter on digital assistance may provide some interesting insights on Mallory's question of how associations should think about these new product and service opportunities.
Mallory Mejias: Do you know of any associations that are actively leveraging AI in that [00:32:00] way?
Amith Nagarajan: I know of quite a few that are working on building exactly this type of vision. I don't know personally of any associations that are already live. With new AI powered products and services. But if any of our listeners are aware of examples that people are out there in the wild doing this stuff, we'd love to hear your feedback.
So shoot that to us through the Sidecar Community.
Mallory Mejias: Absolutely. Where do you expect to see more industry specific coming up in 2024?
Amith Nagarajan: That's a tough question to answer because I think it's literally everywhere. I think the industries that are information driven rather than kind of, interaction, like in person interaction driven, you're going to see a lot of it happen really quickly. Because they're, they're pushing around bits rather than moving atoms.
But I think the industries that are, you know, people centric that require live interaction or moving. Moving physical goods will also see a tremendous amount because there's always an AI. Angle on it or information angle on it. I do think [00:33:00] robotics are gonna advance tremendously in 2024. But putting that aside, just the AI side of it alone, independent of physical movement or interaction. It's gonna affect every industry. So I really don't have a specific one I'd say is likely to be impacted but I think the ones we've been covering here, science, law, accounting are very obvious places where there's going to be massive disruption.
Mallory Mejias: Earlier in this episode, we talked about the idea of the human plus AI, not necessarily AI kind of taking over a profession completely. But in thinking through this topic, I went down the rabbit hole of thinking through industries that will be disrupted for sure maybe totally by AI. And one of those that I think you and I have talked about before, Amith, is translation.
And I am a huge advocate of foreign languages, love learning languages, have spent a lot of time of my life dedicated to doing that. And I think it's hard for me to imagine a world where the, the world of translation isn't disrupted by AI. When we'll see [00:34:00] translations become so widely available so quickly and essentially for free and probably perfect translations.
What, what are your thoughts on that Amith and how can an organization, let's say the association of translators, like how can they be thinking of that and preparing for that?
Amith Nagarajan: That's a great example. And I think, you know, long, long ago, there might have been an association of phone switchboard operators where that entire job is just gone. And so this one might be gone, but for specialized use cases where the translation makes sense to be done by a person because there's a level of art to it, or there's some special scenario where it makes sense to have a human translator, but I think it very much is going to be an area that will be automated.
And I think that this is a net positive in many respects, because right now there's a narrow limitation in terms of where you can deploy your content, your knowledge as an association. Perhaps you're primarily an English language association. And you don't really, you might have a global audience [00:35:00] to a degree, but you're not communicating in the native language of most of your audience if you are a global association.
Even though English is a language that so many people speak many don't. And even the people that do speak English, it's not their first language. And so if you could take away, essentially the tax that they're paying every time they have to listen to you in English or read your content in English you're serving them better than just providing them a single language.
So I think it's an explosion of native content that's going to be available in every language in the world. And that's amazing. That's incredibly great for the outcome. But then coming back to your question of what the you know, the association of translators do. I mean, the question is, is there a shift in the work that translators do?
That is real creative that potentially could retrain the people that are in that field. Could that be the new mission of the association is to figure out new jobs and new roles for those people help them retrain in other areas. Like where do their skills translate to value [00:36:00] add in the world of AI, where the fundamental blocking and tackling of translation 95 percent automated.
Where can these people play? And I think a lot of that depends on like, why are they translators? Are they translators because they just, you know, happen to grow up in a multilingual family and it made sense for them to do it? Were they someone perhaps that just loves the culture of learning different, you know, different cultures and different languages?
And how can you take those people and redeploy them to other jobs? And that's a bigger societal question that we're going to have to answer. But I think some associations are very much going to be in that category where like literally their entire profession. It's likely to go away or maybe be one tenth the size that it is today, and that's an existential question.
Does the association even plan to continue, or does the association no longer have relevance and it should merge with someone else or just shut down? I think it's a valid question to be asked, you know, by the staff and certainly the boards of some organizations. I think for most groups, it won't be as like direct hit on, you know, the middle as the translator [00:37:00] example.
But there's an AI impact somewhere. And so the question is, is do you help your profession embrace AI and leverage it? Or do you refuse it? And, or do you just kind of ignore it, which a lot of people are, I would say in that camp, not so much ignoring it, but, they're aware of AI. But as a practical matter, they're not really doing a lot with it, which to me is functionally equivalent to ignoring something.
If you know about it, but you do nothing. What's the difference? Ultimately, there's someone who actually actively ignored it. So I think associations have to grapple with this, right? You have to look at it as objectively and as brutally honestly as you can. What does it mean for the future of our industry?
Our profession? All this? All this stuff? Can we adapt? Can we take advantage of it? Or does it mean that what we do is going to be radically reduced in the future?
Mallory Mejias: If there was, in fact, an association of switchboard operators, I think that's what you said. It would be really interesting to go back in the past and see kind of what happened to organizations like that in times of major change.
Amith Nagarajan: Yeah, that'd be a fun research project that that we know in AI [00:38:00] that can help with that.
Mallory Mejias: I bet we do. The last topic for today is the New York Times lawsuit against OpenAI. The New York Times has initiated a lawsuit against OpenAI and Microsoft alleging copyright infringement. The heart of the lawsuit is that OpenAI's AI technology, including ChatGPT, was trained using millions of articles from the Times.
Which the newspaper argues constitutes illegal copying. This training, they claim, was used to develop AI products that now directly compete with the Times services. This lawsuit is a landmark case in the realm of AI and copyright law. It raises questions about the methods used to train large language models and the legal implications of using publicly available content for this purpose.
The Times argues that the AI models can generate outputs that closely mimic or even directly replicate its content, potentially undermining its relationship with readers and impacting its revenue streams. The New York Times lawsuit against OpenAI and Microsoft over AI [00:39:00] generated content mirrors Getty Images initial legal action against AI image generators like Stable Diffusion,
but with a notable twist in Getty's approach. While both cases highlight the tensions between traditional content creators and AI generated content, Getty Images has taken a dual approach, which I think is interesting. On one hand, they're challenging the use of their copyrighted images for training AI models that generate similar content.
On the other hand, Getty Images is also embracing AI technology by creating its own image generating platform powered by NVIDIA, recognizing its potential in the creative industry. Amith, what are your thoughts on this lawsuit?
Amith Nagarajan: Well, I think that it is going to be really informative over the next year, two years, however long it lasts to get a sense of, of you know, getting some meaningful case law on This exact issue and the question of what actually constitutes a copyright violation. And so there's arguments that I think are quite [00:40:00] compelling on both sides.
The most immediate surface level reaction to it is if you have an AI that had read a million articles from the New York Times and is able to largely handle whatever the user value needs were, meaning like someone who would have read an article on the New York Times no longer needs to go there and they can go to Chat GPT instead, it is competitive. And so the question, though, is, is this does that still you know, result in copyright infringement? Is that truly what that was or not? And, you know, we're not lawyers here. So, you know, we'll have to defer to the people that are in the field and we'll probably end up getting an IP Attorney on here in the next few weeks to comment on this from within the field. But, you know, my point of view, just from a lay viewpoint on it. The importance of this case is simple that we're kind of in the Wild West right now with AI both in terms of the tech, but also in terms of the legal basis for this technology.
And we need to understand what the ground rules are. There's going to be a lot of movement in the [00:41:00] field around synthetic data using AI generated content to train future AI models, which would potentially reduce the need for copyrighted materials to begin with, I am sure there will be lots of licensing deals.
In fact, I wouldn't be surprised at all if the New York Times, Microsoft and OpenAI decide to, you know, make up and hold hands and, and a lot of money will be transferred between these organizations to do that. But, you know, it wouldn't surprise me at all if that was the case in order to resolve this.
But I think we're gonna learn a lot from this. That's my main takeaway. And I think it's really important that we pay attention for associations. There's a couple things to think about. It's on the offense side of it. If you are a content creator and most of you have your content potentially been illegally used without, you know, obviously without your consent.
And what can you do about that? That's a really interesting question to be thinking about. And then the flip side of it is, how can you defend against this? How can you ensure that your content isn't used or is less likely to be used? [00:42:00] And then finally, the point you made about Getty, both pursuing defense but also pursuing offense in the sense of going after the AI opportunities, you too can do the same thing.
You can use your content as a strategic asset to build new products and services, as we were just discussing.
Mallory Mejias: Yep, I actually went to the, I had no idea about Getty images, image generator. I didn't test it out. I think you might have to request a demo to get access to it, but it's an interesting take for sure to be on the defense, like you said, but then also to embrace it on the other hand.
I'm curious about this point.
I read in an article that one of OpenAI's models, I think it was ChatGPT, in certain scenarios directly replicated like phrases or let's say sentences from the New York Times. Knowing that ChatGPT is a next word predictor, is it solely coincidence you think that it produced the exact same lines of copy from New York Times articles or is there like something else going on there?[00:43:00]
Amith Nagarajan: Well, let's take a quick refresh in terms of how these models work. So when you train a language model or any kind of AI model, you're passing through the model a tremendous amount of training data. And this, this training data essentially is teaching the model a lot of different things. And the model is able to remember elements of what it's learned. But the model isn't storing the content. The model isn't taking the article and storing it in a database somewhere, much like if you're in a classroom setting and you learn something and you remember portions of that. So your neural network is essentially wiring itself up to have long term memories of Some of that information and the AI training process is very, very similar to biological neural networks. And so that's an important reminder for everyone. People assume, since it's a computer that it literally has an exact copy of all the data it was trained on. That is not the case. In fact, a great way to realize that is if you look at the physical size of a large language model and compare that to the training data set, the training data [00:44:00] set is orders of magnitude larger In terms of just the number of, you know, petabytes of disk space relative to the number of gigabytes of disk space that the model typically occupies once it's trained.
The model, by the way, is what a lot of people, you hear the term, the weights. The weights of the model basically is the model. It's the actual files that keep track of, you know, the, the outcome of the training process. And so it's highly, highly compressed piece of information. The reason I point that out is, you know, if it was a simple as like, hey, we copied it and therefore like we're going to use it, you know, our own service.
Clearly, that's a direct Copyright infringement. But in this case, that's not exactly what's happening. It's not deterministic in that sense. And it's, it's, it happens to be that the AI could potentially quote an excerpt from an article that's literally the exact same outcome, but that's just as likely as a person potentially quoting the exact article or an exact subset of an article itself.
So I think it's a really interesting parallel and I'm not sure what to make of it exactly. I think it's, it's a new domain [00:45:00] for us to explore.
Mallory Mejias: Yeah, that leads me into this example that I thought up while I was doing some research. And that is a person who reads history books on ancient Egypt. And let's say this person decides to write essays about ancient Egypt using the knowledge that they gained from those history books. And then that person puts their essays into a book, sells them, earns lots of money.
It's a great book of essays. I feel like you could argue this human was trained on the history books. So, I guess legally, and I'm not saying you know this, Amith, but I don't know. I'm trying to process, like, how this is different when you replace an AI model with a human, given what you just said. That it's not storing exact copies of the information.
It just is not remembering. That's probably not the right word. But is, is trained on it. That's how it learned.
Amith Nagarajan: Yeah. And I think, I think the analogy you're depicting there is perfect because it's exactly what's happening in AI models just at much, much greater scale because these models have consumed large portions of the internet. And so when you think about it that way [00:46:00] you know, can you, the question is, is like, can you reasonably use that analogy to frame the way the legal system should think about copyright in the age of AI?
When we made our copyright laws AI was, you know, nonexistent, obviously. So, are they appropriate? And I think that, in many respects, they probably are. There's, there's probably a really good intellectual framework for deciding what is an original work, what isn't.
If you literally read a history book on ancient Egypt and you copy large chunks of it that go outside of this idea of fair use, which we'll defer to when we get a legal expert on here to really dig into what fair use means in the context of copyright and AI. But if it goes beyond fair use and you literally put it into a new work that, you know, you haven't really added any value to and sell it, you're probably going to get hit with a lawsuit if it gets discovered.
And these days it's easily discoverable, obviously. So, I think that's the question is, is there something novel added to it? Did the human in your example or the AI? And [00:47:00] in what we're talking about here more broadly do anything original with what it learned and produce something different in its outcome?
And some could argue, you know, it's not doing that because it's the next word predictor. But is that that much different from what we do? Is the question. So, I think it's a fantastic and interesting thing, independent of being on either of the sides, right, in terms of being an AI advocate or a content advocate.
I think it's just the type of thing we're gonna have to figure out as a society's move forward. What are the frameworks? What are the legal rules? This also goes to some of our prior conversations on regulation, which is kind of an adjacent topic. You know, what's the framework as a society that we think is appropriate to incorporate into this this new world?
Mallory Mejias: You mentioned this idea earlier of associations protecting their own content and making sure that it has been used in a fair manner and that they haven't been, their information hasn't been infringed upon, but on [00:48:00] the flip side. What do you think are best practices for an association or an individual listening to this podcast to use AI generated copy or images?
Amith Nagarajan: Sure. Well, some association CEOs that I speak with are concerned that if they use generative AI tools, that there's really two concerns that the first concern is that the output of the generative AI tool will not be copyrightable. So they won't have protection on it going forward. And that's one issue.
And then the second part is, are they potentially a target for a lawsuit themselves because the tool they're using potentially infringed upon someone else's intellectual property? Also an interesting concern in the case of the former where you generate something exclusively using AI and there's no value added by a person.
It's likely not to be something you could copyright. That's that was actually an opinion by the USPTO that came out last year that basically said [00:49:00] copyright is intended to protect works of people, not computers. And so the question, of course, then it's still a gray area is if it was partially person, partially computer, can you get a copyright on it?
A great example is our book Ascend. We had a ton of AI help for that. We talk about that in the book. Is a sentence something that we can actually have a copyright on? We have a copyright on it, but is that a valid copyright? For us, we don't really care that much, because our mission with the book is to share the knowledge with as many people as possible.
We're not trying to make money with the book. If people copied it and sent it to everyone they knew, we actually encourage that. We say, hey, download the PDF and send it to everyone. But we're weird, you know, we're kind of in lots of ways, but we're weird in that particular use case. And so, I think that for associations who do want to copyright their work, and obviously we want to copyright some of our work.
It's a concern. It's something you have to think about. So how much value are you adding as a person? And the other side of it is, are you potentially subject to future infringement claims that could come where someone sues OpenAI and also sues you because you're a user of OpenAI's [00:50:00] tools? I think it's a practical matter for most organizations.
The probability of that's pretty low as a fairly small fish in the overall ocean. But nonetheless, there's also protections that Microsoft and OpenAI have offered, and they call it like IP shield or something like that, where they're saying, Hey, if you get sued, we will indemnify you now, whether or not you believe that they will actually do that.
If a lawsuit served in your doorstep is another question. But that's one side of it. And then the other side of it is, of course Ultimately, you know, can you use what's happening around lawsuits and content to create a moat for yourself where you say, hey, our proprietary content we have our own generative AI solution around it.
That is extremely easy to use. It's affordable. It's the best in the world for our domain, and we're going to use the law as a sword to prevent other people from leveraging our content to make their models good. And that's a very reasonable thing to be thinking about, too.[00:51:00]
Mallory Mejias: All right. Anything else there?
Amith Nagarajan: I don't think so.
Mallory Mejias: Alright. Thanks everyone for tuning in to today's episode. We hope you all had a happy new year and holiday season reminder. We are currently enrolling for Sidecars AI Learning Hub. [00:52:00] If you want access to those on demand AI lessons in 2024, the weekly office hours with AI experts, and you want access to that growing community of AI enthusiasts, definitely check it out at sidecarglobal.com/bootcamp. We'll see you next week.
Amith Nagarajan: Awesome.
Thanks for tuning into Sidecar Sync this week. Looking to dive deeper? Download your free copy of our new book, Ascend, Unlocking the Power of AI for Associations at ascendbook. org. It's packed with insights to power your association's journey with AI. And remember, Sidecar is here with more resources for webinars to boot camps to help you stay ahead in the association world.
We'll catch you in the next episode. Until then, keep learning, keep growing, and keep disrupting.

January 5, 2024