Show Notes
Join Amith and Mallory in Episode 7 as they delve into Google DeepMind's groundbreaking AI leap in materials science with the discovery of 2.2 million crystal structures. They'll also discuss the new AI Alliance while breaking down the open source versus closed model debate. The episode rounds off with a conversation around AI governance.
Let us know what you think about the podcast. Drop your questions or comments in the Sidecar community: https://community.sidecarglobal.com/c/sidecar-sync/
Join the AI Learning Hub for Associations: https://sidecarglobal.com/bootcamp
Download Ascend: Unlocking the Power of AI for Associations: https://sidecarglobal.com/AI
Join the CEO AI Mastermind Group: https://sidecarglobal.com/association-ceo-mastermind-2024/
Thanks to this episode’s sponsor!
- AI Learning Hub for Associations: https://sidecarglobal.com/bootcamp
Tools/Experiments Mentioned:
- Betty Bot: https://bettybot.ai/
- MemberJunction: https://memberjunction.com/
- Mistral: https://mistral.ai/
Articles/Topics Mentioned:
- Google’s DeepMind finds 2.2M crystal structures in materials science win: https://arstechnica.com/ai/2023/11/googles-deepmind-finds-2-2m-crystal-structures-in-materials-science-win/
- AI’s future could be ‘open-source’ or closed. Tech giants are divided as they lobby regulators: https://apnews.com/article/ai-opensource-meta-ibm-chatgpt-dd61e99ac8135b36872b3987601067ec
- Who Will Govern the Future of AGI? w/ Emad Mostaque (Stability AI Founder) | X Spaces: https://podcasts.apple.com/us/podcast/moonshots-with-peter-diamandis/id1648228034?i=1000635736854
Social:
- Follow Sidecar on LinkedIn: https://www.linkedin.com/company/sidecar-global
- Amith: https://www.linkedin.com/in/amithnagarajan/
- Mallory: https://www.linkedin.com/in/mallorymejias/
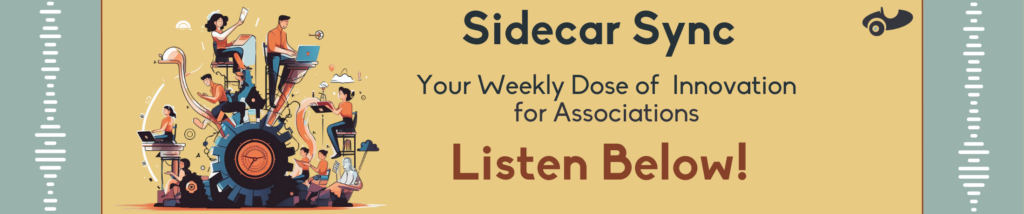
Amith Nagarajan: Greetings everyone. And welcome to the latest installment of the Sidecar Sync podcast. My name is Amith Nagarajan and I'm your host, and I'm here with my co host, Mallory [00:01:00] Mejias, and we're excited to kick off this episode before we talk about all of the latest advancements in AI and how they apply to associations like yours.
Let's first of all, take a moment to recognize our sponsor. For today's podcast. our sponsor is Sidecar's very own AI Learning Hub. The AI Learning Hub is a way for you to get started and continue on your AI learning journey. The idea is that you have access for 12 months to a comprehensive curriculum of AI learning that you can take on a self-paced basis.
That content is continuously updated for you throughout the year. As new advancements in AI unfold and as existing knowledge is updated, you will be alerted and have the opportunity to stay up to date. This Learning Hub also includes live office hours every single week with AI experts from across the Blue Cypress family and a discussion forum to have peer to peer learning and sharing with the rest of the people going through the Learning Hub. To learn more, check out sidecarglobal.com/bootcamp [00:02:00]. And one thing to keep in mind is there are a couple spots remaining for the first 50 offer, which is if you sign up now and you're one of the first 50 people for the Learning Hub, you'll get lifetime access for the same price as a single year.
Mallory Mejias: Those spots are going quickly, so make sure to sign up soon if you are interested in that special offer. Amith, how are you doing today?
Amith Nagarajan: I am doing fantastic. I've been excited about this episode for a couple days. How are you?
Mallory Mejias: I am excited as well. I'm doing pretty good myself. I'm always excited about every episode. But I do feel like this episode in particular, we are diving into some interesting newer topics. I would say for us and all things AI. So I'm really excited to dive into topic number one, which is AI and materials science.
Google DeepMind researchers have made a groundbreaking discovery in materials science by identifying 2. 2 million crystal structures, a feat that significantly surpasses the number of such substances previously known in the entire [00:03:00] history of science. This achievement detailed in a paper published in nature was made possible using an AI tool known as Gnome. The discovery opens up new possibilities in various fields, including renewable energy and advanced computation. The researchers plan to make 381,000 of the most promising structures available for scientific testing and applications ranging from solar cells to superconductors. This venture highlights the potential of AI to accelerate the discovery of novel materials, bypassing years of experimental effort.
The DeepMind team's approach involved using machine learning to generate candidate structures and then assess their likely stability. The number of substances found is equivalent to almost 800 years of previous experimentally acquired knowledge. This discovery has potential applications in creating versatile layered materials and developing neuromorphic computing, which uses chips to mirror the workings of the human brain.
Researchers from the University of California, Berkeley and the Lawrence Berkeley National [00:04:00] Laboratory have already used these findings to create new materials, demonstrating a success rate of more than 70 percent in their experiments. This high success ratio underscores the effectiveness of combining AI techniques with historical data and machine learning to guide autonomous laboratories in material creation.
Amith, why is this important and what does it mean for associations?
Amith Nagarajan: Well, I think it's important on a number of levels. I mean, I find this particular advancement really, really exciting because, you know, we spent a lot of our time thinking about information and in the world of AI, a lot of the effort is focused around really the world of bits, meaning the world of information, that which you can digitize.
And we sometimes forget that there's a whole world. Made up of atoms out there, the physical world we all exist in and materials are everywhere. Materials are part of literally everything we do, everything we, you know, consider doing. And our material science knowledge has advanced over the years, obviously, like all [00:05:00] branches of science.
But this is an area where I think one aspect of our species is that we end up getting in a rut sometimes. And so, you know, you can see. See from the statistic provided that the very first time this particular AI model was used at scale, it developed 800 years worth of research. And we validated in the lab, you know, the last thing you were mentioning is that a very large percentage of these structures are actually considered to be feasible by actual, you know, empirical results in the laboratory, not just from what the AI says. So the point I would make, I guess, is we're finding a way to bring these ideas into the physical world. And this, this particular example is probably the most immediately obvious example, but, you know, we've talked in the past about AI and the context of protein folding.
Uh, and how that affects biology. And there are many other examples like this. I think there's, there's two things I would point out that are really exciting about advances in material science through AI. And you mentioned both of them. One has to do with renewable energy. And [00:06:00] that would be advances in the materials used to build things like solar cells or even you know, more adaptable and flexible materials for things like offshore wind energy as well as the ability to store energy.
So one of the biggest problems that exists with renewables is storage and so battery technology of various flavors. Has some pretty significant limitations at the moment based upon the materials we have at our disposal. Some of those limitations have to do with energy density. Um, right now, for example, the heaviest component by far in an electric vehicle is the battery setup.
And that's because the energy density of lithium ion batteries is actually quite poor compared to gasoline. That's why you can fill up your tank of gas and you can have, you know, however many few hundred pounds of gas that is that you can drive 400 miles, but it takes thousands and thousands of pounds of lithium ion batteries to give you an equivalent range in electric vehicle.
Um, So energy density is one factor that has to do with the chemistry of the, of the battery itself, but also the fundamental structure of it. [00:07:00] And so there's a lot of opportunity to create a new battery technology with advanced materials or novel materials, I should say. And then uh, the other thing that I think is really exciting here has to do with you know, computing.
So computing itself is bounded to some extent right now by our current materials. So we're getting to the point in terms of chips where we're getting smaller and smaller and smaller in terms of the scale at which transistors are etched into silicon uh, to the point where we're running into problems where these transistors are so tiny that, you know, we're running into physical property limitations.
And so in theory, these new materials might help with that. But one of the biggest opportunities around computing has to do with this idea of Superconductivity. A few months ago, Mallory, there was this big news story about something called LK99. You remember that from a few months back?
Mallory Mejias: I do, I remember.
Amith Nagarajan: And that was like a big thing. Silicon Valley was freaking out about it because there was supposedly this material that was [00:08:00] actually discovered a number of years ago by a researcher in South Korea. who claimed that they could achieve something called room temperature superconductivity. The superconductivity is essentially the ability for a material to conduct electricity with essentially zero loss, meaning as I try as I transfer power across like a copper wire.
For any length of distance, there's some loss and you know, it might seem like a small percentage, but it adds up over time. And so the loss lossy nature of conductivity results in a number of problems. One is that actually of the percentage of the power that's generated at say a traditional coal fired plant or even a nuclear plant doesn't really matter what the plant is, a decent percentage of that is lost in transmission on the high energy or high voltage energy transmission lines as well as when it gets stepped down into your local area.
You're losing a lot of power with every additional, you know, mile, foot, an inch of [00:09:00] power lines. And the same thing is happening in chips, where you know, you have semiconductors that have gates or transistors that are turning on and off all the time. And as all this is happening, you're losing a tiny bit of electricity through just the process of the conduction itself.
Superconductors essentially right now the only superconductors we know of are required to be at extremely cold temperatures, so they're not really practical in applications like computing or power transmission. But if we have a room temperature superconductor that means that we would have a different material to use to both transfer power over long distances, which would mean higher efficiency.
It would also change actually the entire nature of the of the grid because right now power has to be generated pretty close to where it's consumed because so much of it is lost over time. You can't really easily say, oh, well, over in Arizona, we have tremendous solar potential. Let's pipe that over to Texas or pipe that over to North Dakota.
The grid is national, but largely the consumption of [00:10:00] electricity is within 50 miles of where it's generated right now. So it has that impact as well. But back to computing you know, in data centers that house all the GPUs that run AI as well as just traditional computing, the biggest cost has to do with cooling and the cooling costs are high because energy dissipation from the chips is so dramatic that it results in the whole room, these massive rooms of computers being extremely hot. So you have to do liquid cooling and air cooling. Some people are even putting these data centers like in places like, you know, far, far north where it's they can pump in cold water from the sea or from ice and things like that. So the impact is potentially massive across all these areas.
And ultimately, with computing, if you have superconductivity you can build different kinds of chips. You have opportunities for new form factors, new physical approaches. And that all maps out to a really key point, which is this breakthrough material science will lead to an exponential curve accelerating in material science, [00:11:00] which will in turn come back and further compound the already exponential growth in computing and artificial intelligence.
So. It's a big deal.
Mallory Mejias: What happens when you mix two exponential curves together? What happens then?
Amith Nagarajan: You get an even faster exponential curve. And, you know, we're seeing exactly that happening right now. There's this convergence of multiple distinct exponential curves. And we've actually had that happening in the world of material science around solar power for over 40 years. There's been, you know, the equivalent of Moore's Law happening with solar cells.
The cost of generating electricity through photovoltaics has been dropping roughly every 16 months for 40 years. And that's why solar is now actually a pretty reasonable option for homeowners, why we can do solar at scale. But that's happened primarily through economies of scale. As production has radically increased, that drives costs down.
But the solar cells themselves are essentially awaiting for a radical breakthrough that increases their efficiency. You know, current production solar cells capture about 25 [00:12:00] 26 percent of the actual energy that they're receiving. So when, you know, the sun hits the solar cell, the cell is only capable of capturing around 25 26 percent right now.
And new materials are actually capable of taking us to a completely different level. If we can get to 30, 40, 50 percent efficiency in capturing that energy. It's also a game changer in that area of renewables. So that's also exciting.
Mallory Mejias: So with all these big changes and potential benefits from having a room temperature superconductor, for example, I imagine this is something that we have been looking at for many years. I'm understanding you to say that a tool like Gnome could create a superconductor because it's kind of like a compilation of the past 800 years of scientific discoveries in terms of material science.
It hasn't done that yet, right? But it, it's very, it very easily could.
Amith Nagarajan: Well, I don't know how easy it would be. I think that, you know, in concept, yes, it would be easy over time, just based on the sheer [00:13:00] scale of it. Because if you think about Gnome, actually, it created the equivalent amount of research of the last 800 years in a very short period of time. But it's actually novel materials.
That's the interesting thing about it, is unlike something like a language model, which essentially is using the patterns it's learned from all of its prior training data, Gnome is actually creating novel materials, and it's a different approach to machine learning than what powers, let's say, ChatGPT.
But the really key thing to understand is that it presents more ideas, essentially. It presents ideas for materials, which we then, of course, at the moment, have to go and synthesize them in the lab, make sure that they actually work the way the I theorizes that they'd work. And as you said earlier, and I reinforced, it's like 70 percent so far of the experiments have been successful.
That doesn't mean it will maintain that rate, but 70 percent is pretty darn good because, you know, when a scientist has a hypothesis about a new material you know, the probability of getting anywhere close to that is much, much lower, right? So, and then that [00:14:00] in turn is an unlock because if we have more candidate materials, then we have more that we can test against these potential applications like a superconductor or you know, new battery elements and things like that.
So. It's not that this particular AI breakthrough will result in a superconductor. Necessarily. It just makes it way more likely that applications like that that previously have been considered the realm of perhaps science fiction or just far out there are coming much, much sooner.
Mallory Mejias: What could this mean for associations, particularly material science organizations or scientific organizations?
Amith Nagarajan: Well, I think it's, it's an incredible opportunity. So people that are in material science or by extension, if they're in any branch of engineering frankly, any branch of science, like this particular innovation is relevant to everyone because you have to look at it from the viewpoint of what does this mean for scientific discovery?
What does this mean for applications of these? materials and engineering of various flavors. And the answer is, is it means a lot. And you have to be at a minimum aware of [00:15:00] this stuff and what it means for your members, for your audiences. What kinds of information are they going to be looking for?
Your organization, even if you're adjacent to a market where materials are super important, you have to be up to speed on this stuff so that you can speak about it intelligently so that you can curate the right content for your communities so that you are not only staying relevant, but like really advancing the field.
You have a responsibility to do that. So I think people that are like right in that space, this is clearly in their wheelhouse. They've got to go figure it out. Um, but I think all associations should pay attention to this, even if you're in a field seemingly as distant as, say, the law. If you're a bar association, why would material science innovation like this matter to you?
And the way I'd recommend thinking about it is that it's essentially a domain specific AI advancement. So here we're dealing with something that's deeply scientific. But what happens when you apply AI to a very narrow problem is quite interesting. Whereas the AI we spend a lot of our [00:16:00] time thinking about talking about in this podcast and in the association community generally, is quite wide in its potential application. You think about Chat GPT, it's a language model. It's very general purpose, whereas Gnome is something you or I probably will never use because it is hyper specific to this problem, and it solves this particular problem in an incredible way. Um, so the way to think about this, if you're, say, a bar association or an accounting society is what can we do in our domain with a narrowly focused AI approach for our specific set of problems?
Because there are ways of solving all sorts of problems if you focus an AI solution on your domain. So that's really the thing I would suggest taking away if you're in a field that seems like you're far away from this. Because AI that's domain specific can go far, far faster and deeper than the general-purpose AIs that everyone is using right now.
Mallory Mejias: As AI continues to revolutionize fields like material science, what steps can associations take to prepare their [00:17:00] members for the changes and opportunities that arise from such advancements?
Amith Nagarajan: Well, you know, I think the most important thing to keep in mind as all of this crazy pace of AI innovation continues is that you’ve got to start somewhere. You have to start learning and educating your team and your board and your volunteers. And it's interesting, too, because I talked to a lot of different association leaders, CEOs and others.
And, you know, the folks that are in technically oriented organizations. So if their members are scientists or engineers or doctors, it's interesting because some of those folks tend to be a little bit more hesitant at introducing AI or talking about AI with their professions because they kind of have this assumption that those people are so deeply technical that they don't really need the association to talk to them about AI. But I find that to not really be the case. You know, I find people even in computer science, for example, who are not up to speed on AI because [00:18:00] they're deep in their rabbit hole. They might be really great at a particular branch of computer science, for example, that has very little to do with AI. And they know very little about AI. So I think that's one thing that we should step back from and revisit our assumptions essentially and say, do we believe that it's not our role to talk about AI or to lead the AI conversation in our community because our audience is technical in nature? And I would encourage you to challenge that assumption.
You may be right in having that assumption. If you're the AI association, maybe that's the case. But, you know, realistically many of your members are probably looking for leadership in this area. And for you to be a leader in this area, you do not have to be the AI expert, but you have to be educated.
You have to be aware of artificial intelligence, its capabilities, and you have to stay up to date. It means there's more work to do. You've got to take advantage of this stuff in your organizations as practitioners, because if you just learn about it theoretically, you're not gonna really understand it.
You have to put it to use a little bit. So that's always the first starting point. And then, as far as, like, [00:19:00] specifically this type of evolution that's happening with Material science and other and other similar things. We're bound to hear about in the near future. I think it's critical that associations start developing a content strategy.
Around advancements in their field, but also adjacent fields, associations tend to be like, you know, most of them anyway tend to be a mile deep in an inch wide versus, you know, the mile wide and an inch deep where you're super broad and not super deep. Some associations are like that, but most associations tend to have a very narrow niche.
And they tend to hyper focus their content strategy on that niche. I think it's important for you as an association to consider kind of pulling your head up a little bit and say, hey, what else is happening in these adjacencies? And what's our role? And perhaps in collaboration with other associations to advance knowledge in our domain.
But with the idea of that context and the world around you and all the changes that are happening. So those are a couple tips I'd provide to association leaders right now [00:20:00] and how to think about this particular advancement.
Mallory Mejias: That's a really great point, Amith. My fiancé is in PA school, physician assistant, or soon to be physician associate based on the work that AAPA has done for the name change, but he is itching to learn more about AI in the context of healthcare and specifically how it relates to PAs and the future of the medical profession.
It's a great point, because I don't think he looks to AAPA, for example, as the organization that should know every single thing about AI and the healthcare profession, but he does look to that organization as a place where he can find that community, to find other members who are interested in the topics, and then looking to that organization to be kind of a leader in that space in terms of curating content so he can learn more about that.
And I think it's a really interesting point that even if you do have a more technical membership, that you can still be a leader in that space. But first and foremost, you need to be educated.
Amith Nagarajan: Yeah, Mallory, that's a great example. And I think there's so many fields just like that, that people are hungry [00:21:00] for the information. And you gotta remember, your members are busy. You know, these are folks that are out there working hard. Either they're studying to enter the profession or they're working hard in the profession.
They're doing what they do. And they probably have very little time to learn about AI and even think about how it's applied. You know, one thing I'll mention, it's a bit of a plug for Sidecar, but a lot of people don't know that Sidecar actually can partner with your association to develop a member facing AI Bootcamp. You know, we talk about AI bootcamps a lot here because they're so important to help you get started and keep going with AI and Sidecar does offer a custom bootcamp service where the sidecar team and experts from other companies within our family will build a curriculum specifically for your audience and then actually execute that bootcamp, developing the content and delivering it.
And that's been increasingly popular in recent months as associations think about how do you blend the AI expertise with your domain knowledge. So just keep that in mind. There are lots of ways to approach this, but I would encourage you to think about [00:22:00] how to bring AI knowledge to your audience as a high priority as you enter 2024.
Mallory Mejias: I'm really excited about our next topic, which is open source versus closed and the new AI Alliance. The tech industry is currently divided over the approach to AI development, particularly between open source and closed models.
This debate has significant implications for the future of AI involving key players like Facebook's parent company Meta, IBM, Google, Microsoft, and OpenAI. Meta and IBM have launched the AI Alliance. Advocating for a quote unquote open science approach to AI development. This stance puts them in opposition to Google, Microsoft, and OpenAI, who favor a more closed model.
The AI Alliance, which includes major tech companies and universities, emphasizes the importance of open scientific exchange and innovation in AI. The core of the debate lies in whether AI should be developed in a way that makes the underlying technology widely accessible. [00:23:00] aka open source, or more restricted aka closed.
Open advocates like IBM's Dario Gil argue for a non-proprietary approach, while others express concerns about safety and commercial incentives against open source AI. OpenAI, despite its name, develops AI systems that are closed. The company's chief scientist, Ilya Sutskever, highlights the potential dangers of a powerful AI system that could be too risky for public access.
This view is contrasted with the open-source philosophy, which has been a longstanding practice in software development. The debate extends to regulatory and ethical considerations, with different tech leaders lobbying for regulations that align with their AI development models. The US and the European Union are actively working on AI regulations, considering the balance between innovation, security risks, and ethical implications.
Amith, what are your thoughts on this open-source versus closed debate in the alliance? [00:24:00].
Amith Nagarajan: Well, I’ve got a lot of thoughts on this topic and I’ll speak a little from personal experience on this as a software developer and someone who started software companies for the past 30 years. I think there’s a place for both open-source and closed models. So I think that in some cases, it makes sense to have proprietary or closed source software. And, I think that in the case of AI, it's a super interesting debate because the people who are believers in proprietary or closed source technology, which includes OpenAI, even though their names is open, that they are not an open source company and where they actually, to be fair to them, they do have a couple models that are open source, but most of their products, the ones that you're familiar with are not.
It’s interesting because there's a whole community of people who are arguing the open source is the safe way to do AI. And then people are opposite. People are arguing the exact opposite, like Ilya. At OpenAI and the rest of that team. And here's my take on it. I think that, To the degree that you think you can control the technology[00:25:00] you could make the argument that safety mandates closed source. So if I believe that my company solely can actually control the rate at which AI is developing, then it does make sense for me to consider that as a way of containing it. And containment is a strategy. I don't particularly believe in it being a good one.
And I know we'll talk more about that later in this podcast under a different topic. But containment is the concept of saying, hey, we're going to literally put this thing in a box and somehow control who can do what with it when and how and the open source community is saying that's not realistic.
The genie is out of the bottle. People are developing these things at a crazy pace. Just the models we know about, there's hundreds and hundreds of models being developed, many of which are out there available for use. And so the question is, like, can you really contain this thing at all? And then, of course, there's questions of motivation.
Why is it that the closed source or proprietary community is arguing for [00:26:00] regulatory Oversight and open source community, by the way, is not against that. It's just the question of what's the practical approach to regulation in an open-source world. But perhaps, you know, some of the folks I'm not suggesting open eyes in this camp, but perhaps some of the people that are arguing for closed or proprietary models stand to benefit from regulatory oversight of models.
For example, if you're the leading player or one of the leading players that has a ton of capital and you find a way to help support regulation that comes in place and mandates, let's say government approval for every new model that helps you a lot as the incumbent because you're the leader in the space or you're one of the top five leaders in the space.
I'm not suggesting that the people who are arguing for this have a hidden agenda. I'm simply saying that that's a fact. If you are successful in arguing for regulatory oversight and you're a leader in the space, it benefits you. So we have to take that with a grain of salt and just at least consider that as a factor.
Not necessarily the motivating reason [00:27:00] behind why these companies are arguing it. Perhaps some believe that to be the case. I'm not suggesting that myself. Ultimately, you know, I believe there's tremendous value in open source in the world of AI, and here's why. I don't believe that containment can work.
I believe that AI has to govern AI, ultimately, because nothing else will keep up with AI. So, and there's a lot more to unpack with that statement, but I believe that open source is a critically important part of the solution. It also is about accessibility and being able to make the AI technology benefit all of humanity, as opposed to a very narrow slice of humanity.
And I believe open source is far better positioned for a number of reasons to do exactly that.
Mallory Mejias: What about this counter argument, though, that it's just too risky right now for the public to get access to it not knowing what people might do with these kinds of [00:28:00] models.
Amith Nagarajan: I agree that it’s incredibly risky and I’m worried about it but I don’t think that it’s a practical debate because the technology is out there. The technology is already out there to do a lot of damage and, you know, I think there’s a lot to be concerned with there. I also think there are a lot of ways to make things safer going forward. We can also try, you know, for example, the AI Alliance is going to have standards, they’re going to have things for developers to do before releasing models, but ultimately, you know, I think there has to be when you think about governance or safety in the broader sense, like from a societal perspective in terms of safety, you have to think about how do you know when these things are doing bad things because someone can take a model and even if it's like a last quote unquote last generation model. So, for example, GPT 3.5. Up until March, it was the state of the art best model.
Everyone was worried about safety with GPT 3.5. Now, there are a wide number of open source, free models you can download and install and literally get working on a fairly small computer that are GPT 3.5 capability level. And this is now December. So in nine months that's happened, right?[00:29:00] Now GPT 4 right now is in its own league still.
Gemini from Google is supposedly going to be at or better than GPT 4 in certain categories. It'll also be proprietary or closed source. Currently none of the open source models are as generally good. As GPT 4, but some of them are as good at GPT 4 in certain subcategories, and they're already open source.
So I guess the point I'd make is what we have to be focused on societally is how do we actually monitor and govern these things at scale? And to me, I don't know what the solution is, but the only solution I can dream up of being possible of handling that is an AI powered solution. So that's where I'm coming from when I think about open source, I also think open source has a really key advantage, which is, first of all, you know what the inputs are, you know what you've been doing training the model on, you know what the code is, which the code, by the way, in these neural nets is incredibly simple. It's usually a few 100 lines of code, and then it's open weights, meaning that the actual weights that define what the neural network is are open.
[00:30:00] Now there's this movement around interpretability is what it's called in the research field, which is basically to say, hey, how do I understand, like, how this model is constructed and what it's actually doing? It's in a way, like, almost like doing a brain scan on a person looking at which neurons light up when different stimuli are entering into our, you know, into our range of vision, for example.
And we're doing similar things in AI research right now to understand how these models actually work, so they're less of a black box. But you can't do that with a closed model. With open-source models You can do that freely and the research community can really dig into these things and understand how they work.
And that's a critically important element of advancing AI safety. And closed source companies have to do that themselves. They're limited to just their own capabilities. And even if those are vast, again, their agenda might be different than what the public is interested in.
Mallory Mejias: This next question might be obvious for some listeners, but I had the question myself, and I know we have some less technical listeners on the podcast. So I wanted to ask it anyway. I thought GPT 3.5 and GPT 4 were open source [00:31:00] because I know developers can use those models and the products that they build. Can you explain why they aren't and then what open source actually means?
.
Amith Nagarajan: Sure happy to, that’s actually a great questions because I think a lot of folks get confused by that. So it used to be, in the software development world, that a company would vertically integrate it’s product meaning that it would build all the layers of technology that was needed for the value or the consumer of the product, and this is true, even as a common thing, 10 years ago. Think about like ERP. Systems or AMS. Systems. There weren't really APIs for these products in a general sense. Some companies had them, but for the most part, you know, you use that company's complete tool kit top to bottom. And then what started to happen is, you know, people would release APIs which would allow people access to their data to their application functionality and other programmers. Other developers could work with APIs so that creates access, as you're pointing out. But it doesn't mean it's open source. So [00:32:00] you can, as a developer, anyone can go to OpenAI. com, sign up for a developer account, get an API key, and start writing code against their API.
The difference is, open source means you have access to the actual underlying software code. The code, and you can run it on your own computer. So, you can say, hey, I, I really like that. I'm going to take that open-source code, and I'm going to run it myself. And, depending on the type of open source license you can do that, you know, for free.
There are some open-source licenses that are intended to be respected, like for research purposes only. But generally, open-source licenses allow you to do pretty much whatever you want. And you can even modify that code. So, you know, if we have an open-source project, like Member Junction, which is a new product that's being released to the market as an open source common data platform for the association community member junction is totally open source. So the actual software code itself is being published and anyone can download it. Anyone can modify it. Anyone can actually turn it into their own commercial product if they wanted to, even though [00:33:00] MemberJunction itself.
Uh, is a not for profit dot org site that has free software for download. People could create commercial products with it, and so that's a true open source approach. The best examples of open source, though, would probably be Linux, which is the Unix style operating system that's, you know, runs by far the majority of the infrastructure for the Internet.
And also WordPress, which is the most popular content management system on the planet running, I think, over a third of all websites run on WordPress, which is totally open source.
Mallory Mejias: I'm wondering, too, how this divide between open source and closed AI models impacts associations, particularly, do they need to choose a side right now? Do they need to pick one side of the alliance or the other? Or can they just be open to using the models that best serve them?
Amith Nagarajan: I really like the latter option because I think of it more from the perspective of building an architecture, both in your business architecture and how you think about it and then in your software architecture where you have extensibility and what a lot of people call [00:34:00] plug ability or the ability to like plug and play different models.
And so it's really important not to hard code yourself to a particular model or a particular company. That gives you more flexibility in your business model. It gives you more adaptability, and it also there's less vendor lock in. So and there can be, by the way, there can be vendor lock in essentially with open source, too.
So if I just go and say, hey, I'm gonna take, for example uh, something like Mistral is an open source AI company. They're in Europe. They just raised 450 million euros. Uh, And they have a 7 billion parameter model, which for those not familiar is a very, very small model. It's 100 the size of or less more than 100 the size smaller than GPT 4 in their 7 billion parameter model.
And it's actually as capable as GPT 3.5 in many respects. They just raised a bunch of money. They're an open-source company. And so I wouldn't suggest to you just because Mistral is open source that you should say, hey, I'm home free. Let's go build my entire software infrastructure around Mistral.
[00:35:00] Specifically, I wouldn't recommend that. I wouldn't recommend that with any particular product. I would recommend building a layer between them so that you have this degree of pluggability and you can swap out these models over time. It's not literally as simple as plug and play. But it makes your life a whole lot easier, and it's one of the big, big problems associations suffer from with their traditional IT, like AMSs and CMSs, is that there's this horrible vendor lock in that occurs, and then you're like frozen in time, basically, and you can't do a whole lot.
So, I think associations need to be a lot smarter about the AI stuff they're implementing, and put that layer of installation in between the model and whatever applications they want to put in place.
Mallory Mejias: The next topic we are covering today is AI governance. Amith, I think this will be an interesting conversation for sure. This topic was inspired by a conversation between Peter Diamandis and Ahmad Mustaq, CEO and founder of Stability AI. I'm going to dive into a couple of key points from that conversation about AI governance but we will be linking the full [00:36:00] conversation in the show notes. If you want to give it a listen.
Number one, Ahmad emphasize the importance of distinguishing governance from safety and alignment in AI. When the end goal or direction of AI development isn't clear, there's a tendency to overly control or halt progress due to potential risks. However, if you have a clear objective, safety measures can be more effectively aligned with that objective.
Number two, the idea of containing AI within certain boundaries is becoming increasingly impractical as AI technology advances and becomes more integrated into various aspects of society.
Number three, most safety discussions around AI have been centered on the outputs it generates. However, there's growing recognition of the importance of considering the inputs, particularly the data sets used for training AI models. Along with that, there's a call for establishing standards for the data sets used in training AI models. This is crucial because the knowledge and capabilities of AI models are directly influenced by the data they are trained on.[00:37:00]
I think it was Ahmad in this conversation who gave the example of anthrax, I believe. And he said, if an AI model hasn't been trained on how to create anthrax, it won't know how to do that.
And number five, Ahmad advocates for each nation to develop its own AI model and grant ownership of this model to its citizens, suggesting a more democratized and localized approach to AI development and governance.
A lot of key points from this conversation, Amith, but I want to dive into that first one, particularly about governance and then safety and alignment on the other side. How can associations approach that balance and what's your perspective there?
Amith Nagarajan: Well, I think this is a fascinating discussion. And you mentioned that the show notes will include a link to the full pod episode between Peter and Ahmad. And I think that'd be a worthy listen for everyone of our listeners to check out if they want to dive deeper into this topic. It's a fascinating discussion.
You know, I think that it's really important to think about it from the perspective of [00:38:00] that goal setting. And so governance is about achieving goals essentially. In a sense, it's like it's an oversight function, but it's an oversight with a particular goal in mind. Like it's governance isn't this like nebulous thing, like you have to have an objective function or an objective that you're seeking and safety and alignment obviously is, it's kind of a similar topic, but to the point that he's making. It's not so much about like stopping progress. It's about kind of looking at the overall measurement of what you're doing along the way. And to the point that I've made a number of times in this podcast and writing and what Ahmad says is that containment isn't really an option.
So it's more about like understanding where things are and then being able to kind of, I wouldn't even necessarily say monitor where you understand what's going on, but like to have degrees of monitoring that are there in order to have a sense that you are governing something. Um, So that's part of it.
And so part of that's the macro perspective of what's happening in the world. And Ahmad talks a lot about, like, AI equity, and I think the work that [00:39:00] he and his team at Stability AI are doing is fantastic around that. A little bit about them, actually. The Stability AI, they're the people behind the stable diffusion model, which is similar to Dali and mid journey, and it's an open source model. It's one of the most popular models. It's been downloaded millions of times by developers, and they have a whole bunch of models that are coming out. They're doing text to video. They actually have a language model they're working on, and their goal is to have models for every major use case.
And then to his point, trained on localized data for each country because the culture, the norms, obviously the language, it’s different in each locale, and so it's important to consider that as well. But I think we can learn from this in the sense that the association can kind of take a page from that book and think about their association almost like a nation and say, hey, What do we need to do in thinking about how this applies to us? You kind of, you kind of are your own nation, your own domain. And so can we learn from that? Can we really carefully think about our models and potentially think about those inputs? He talks about so [00:40:00] eloquently in that podcast about how those inputs drive what the model does and then potentially think about that in terms of how associations consider safety and alignment if they're using high quality data relevant to their domain to train the models that they're using, will those models be better from a safety and alignment perspective than general purpose models or some combination? So I think there's a lot to unpack there.
Mallory Mejias: What's your perspective on data standards around the input that we're putting into these models?
Amith Nagarajan: Well, I don't know that this, the word standard is, is kind of a complex word in that it can mean different things to different people. It could mean the format of the data and how you feed it in so that it's easier for models to share training data. That, I think there's certainly worthy standards being developed around that.
That type of standard. But the standard of the content of the of the data being passed in, I think that's where Ahmad’s point would be. That's needs to vary based upon each like use of the model or each organization or each country. There's an important point. I think let's draw the analog [00:41:00] back to how we learn as people is that, you know, if you go to a school that doesn't teach you a particular subject, you're not going to know about that subject. So in the case of like, bioweapons, of course, if you don't know anything about biology, then you can't create a bioweapon. Now, of course, you know, there's, there's dual use for a lot of information, right, in life. That's true for language. Language can be used to help people and it can use to harm people.
And so, so is the case with most other disciplines. And so, I don't know that the training data can be limited to the extent where you can truly make a safe AI exclusively by controlling the input data. But that's not really what he's saying. He's saying that that's part of the solution. And so far, the solution around alignment and safety has been mostly focused on taking the model that you've generated based on these vast amounts of data without much consideration to what these inputs are.
And then you essentially try to prune it on the back end. So you've sent your kid to school at a place where they've learned who knows what. And then after they've gone to school, you say, [00:42:00] hey, Johnny, don't make a bomb, right? As opposed to, like, don't teach him how to make it in the first place. And that's like a radically simplistic version of the whole problem, right?
Because again, lots of information is dual use, but I think that it's an important part of the conversation that he raises that isn't considered enough in the in the AI safety circles. It's mostly about hey, you have a model. How do you make that model safe? And there's a lot of work that's really critical there because of the subtleties, because you could say, hey, well, actually the same knowledge to make a bomb is necessary to understand chemistry and physics.
And if you want scientists coming out of this thing or scientists to be able to use this model, then you have to have that knowledge. So there's a lot there. And then again, ultimately these tools are going to exist in safe versions and then people are going to create like, you know, the uncensored unsafe versions.
Those models exist right now, by the way, in the open-source community, and there's some for profit vendors that have models out there. That are not censored. I mean, just think about what X.ai is doing with grok, you know, that's like a version of Chat [00:43:00] GPT. It's like not nearly as capable as GPT 4. I haven't used it myself, but you know, that's kind of the point they're making is like hey, we don't want everyone to have to play, you know, kind of nerf games. We believe that it should be more, you know uncensored and whether you agree with that or disagree with that they're doing it and that's also true for these uncensored open source models.
So once again, on this topic, I don't have an answer. I'm not suggesting that I know what the right solution is, but I do think Ahmad calls out some really important things, that the inputs are as important, and his argument is perhaps more important than the outputs. And we have to really focus on that when we think about how we deploy AI.
Mallory Mejias: It seems Ahmad is also suggesting that we have the right to ask these kinds of questions about where that data is coming from. He gives another example that I thought really resonated, which was if we have someone teaching us about a topic we know nothing about, let's say material science, because that's what we've been discussing today.
And they're teaching you all these things and telling you all these things. You have a right, I think, to ask, well, where did you go to school? Did you take courses on material science? How did you get that education? [00:44:00] And he's advocating that we should be able to ask those same questions of the models we're using.
Where did you get that information? What kind of inputs went into this? And I think that's a fair point.
Amith Nagarajan: I agree completely. I love that part of the discussion. I think it really conceptualized it in a way that people can relate to, because if the model is trained on this mystery sauce, you don't know who made it or where it came from. Then how can you expect to have a clear understanding of what's going to come out of it?
So I think there's gonna be way more transparency around training data going forward than there has been historically, which is exciting. I think it's really important, but that kind of goes towards the whole idea of open source. Whether it's open source or open training data or open weights or whatever elements you want to open up, the more we create openness, the more visibility we have, the more interpretability we can have, the more we can actually learn as a community to but yeah, I think that that particular example is really powerful because, you know, if you're gonna go hire an employee, it would be pretty reasonable to ask them where they went to school.
And you're hiring an AI. I mean, that's, that's the way you need to think about [00:45:00] it. The AI is something you're hiring, and so you need to know that it's been trained in a way that you agree with.
Mallory Mejias: The idea of every nation potentially having its own AI model is interesting to me, and I immediately started thinking about every association having its own AI model in the future. Do you think that's the path we're going down?
Amith Nagarajan: I think the, the national boundary thing is really interesting. I, I certainly think for larger nations that have the resources to do this at scale, it's an interesting resource to think of, like. Almost like a public utility that that nation has this great base set of AI models that everyone can use.
Maybe they're free and funded by taxes. Maybe they're very low cost, but you almost like think of them like the way you think about public utilities and you want to make electricity and communications available to every citizen at low or no cost to ensure equity and distribution and all that. And AI, unlike electricity and communications, has an opinion about things because it's a much higher order type of you know, capability.
And so like, if you train one [00:46:00] model on American history textbooks, and then you say, let's take that model and have it teach grade school kids in another country, will that be appropriate? Will that align with their perspectives, their culture, their norms? The answer is probably not, right? And so part of what he's arguing for is that, you know, you, you have to have this degree of localization in order to have cultural alignment and of course, this means some people will argue. Well, of course, you know, some of the despotic authoritarian regimes that are out there will use AI to further enhance their control and speed propaganda into the brains of their people. And of course, they're going to do that.
Unfortunately, that AI is not a solution to that problem. But you know, for countries that are free and open and democratic, you know, this becomes it's not that conversation. It's more of the conversation of cultural norms and respect and honoring the traditions of that locale rather than saying, oh, well, because you know, the U.S. for example, at the moment has a substantial, you know, share and lead in this particular category. Our model should power the world with all of our training data. And the answer he's saying is clearly not. And I agree with that. I think there's a degree, a really [00:47:00] strong argument necessary to be made around that.
Now, does that mean you have to have entirely different models for every country? Or could you fine tune a model? Fine tuning is this process where you take a base training for a model that's done, which is the very, very expensive, computationally intensive thing that costs in some cases, hundreds of millions of dollars.
You do that once and you have your base model and then you fine tune it for each of these regions or locales. I think there's a lot of potential around that as well, rather than having to rebuild the model from the ground up in every country, and I think particularly there might be something where let's say all of the nations in Europe might agree to some base standards.
But in Greece versus in France, there might be a different model. So there's a lot of opportunity around it. And then coming to the second part of your question, Mallory, in terms of associations and other organizations having their own models I think the probability of an association doing complete pre training from scratch of their own totally custom model is pretty unlikely except for the largest associations who have a need to do [00:48:00] that if they have like just a massive, massive repository of content that justifies the creation of a hyper specialized model, they might do that. But I, I think that most of the general purpose models can be used as a starting point. And then perhaps there will be a fine tuning layer on top of that, where the association's content, its rules, whatever else it wants to bake in is put in. And there's ways that there's multiple technology approaches to that fine tuning is a particular term of art in the AI world where you're doing more training on top of the base training, and then the model itself understands this additional knowledge, whereas there's other techniques that are available as well to essentially create the same outcome of grounding an AI in your content or in your methodology. So there's a lot of approaches for that.
I do think AIs that are association specific are very important. We talked about that a lot in the AI book we released earlier this year-Ascend. That book is available for free to download at sidecars website as well. It's sidecarglobal.com/AI. [00:49:00] And in the book, we talk about this idea of an association digital assistant.
And the idea behind the digital assistant is that it's an expert in all things your association. It knows your processes, your policies, your content, your events. It knows basically everything about your association. And through that knowledge, this digital assistant is able to provide a very different level of value than ever previously even conceived to your members and to your audience at large.
And that that's a really exciting concept. You can't do that with Chat GPT. You can't even do that with a custom GPT. You have to really tune the experience and make sure that it's accurate, that it's truthful, that it also aligns with your values and with your language and your tone. So there's a lot of work involved in that, but the promise is extraordinary.
So I would definitely encourage associations to really consider that in their road map. Think about where does an association digital assistant fit into your future?
Mallory Mejias: Yep. I think that's exactly right, Amith. I was thinking more so of fine tuning a model and every association having its own fine-tuned model. Could you [00:50:00] say that a tool like Betty Bot is exactly that, a fine tuned model for an association?
Amith Nagarajan: Yes, conceptually, that's exactly what Betty Bot is. Betty Bot uses a number of different underlying techniques. Betty Bot can have a fine-tuned model. It can also use prompt engineering and other techniques that are available to essentially create the outcome, which is that Betty then is specifically tailored to your association's content. Your style, you know, your tone of voice, things like that. Uh, your norms, your values. Betty can be taught essentially everything about your association and then provide you that digital assistant. And that's one of several ways to approach it. And fine tuning is one of the techniques that Betty is able to use on your behalf.
You don't actually have to go and do the fine tuning work yourself because that's a pretty involved technical process.
Mallory Mejias: Amith, what steps can associations take now to prepare for future challenges in AI governance?
Amith Nagarajan: Well, I definitely would recommend checking out the podcast. The link to you know, with Peter and Emma. That was a fantastic episode. I would encourage people to read about governance and [00:51:00] AI safety as well. But I think, you know, the key is you can't govern that which you do not understand. So if you want to have a chance at governing the AI that's used in your organization or around your association's domain. You have to first start by learning a little bit about AI. So get started on your learning journey. Get going. Find ways. There's tons of resources out there. Obviously we have a bunch at Sidecar, but learn how AI works at a high level. Get started experimenting and then you have a chance of actually having an opinion that you can implement with your governance strategy.
You can't really govern something if you don't really understand what it's about.
Mallory Mejias: And I wish I only knew of a place where people could go if they're hoping to learn more about AI for associations, which is a great segue and plug for that Sidecar AI Learning Hub. Reminder everyone, if you want access to those flexible on demand lessons that are regularly updated. Side note too, we actually just added a brand new lesson on Haygen.
So if you're interested in AI for video creation, you should definitely sign up for the AI Learning Hub so you can watch that lesson. If you [00:52:00] want to have office hours with access to live experts weekly, if you want to have access to a community of fellow AI enthusiasts, I highly encourage you to sign up for that AI Learning Hub.
And reminder, we have a couple of spots left for that special offer, which is a lifetime subscription for the price of one year. So definitely check that out at sidecarglobal.com/bootcamp. Amith, thank you so much for the conversation today. I'll see you next week.
Amith Nagarajan: Thanks very much.
Thanks for tuning into Sidecar Sync this week. Looking to dive deeper? Download your free copy of our new book, Ascend, Unlocking the Power of AI for Associations at ascendbook.org. It's packed with insights to power your association's journey with AI. And remember, Sidecar is here with more resources for webinars to boot camps to help you stay ahead in the association world.
We'll catch you in the next episode. Until then, keep learning, keep growing, and keep disrupting.

December 11, 2023